In the Episode 40th live session on March 15th, 2024, we delved deeply into the realm of deep learning AI. We explored the fundamentals of deep learning, recognizing its significance as a breakthrough in AI applications.
Deep learning AI, a subset of artificial intelligence (AI), has rapidly gained prominence since its inception. Initially introduced and popularized in the early 2010s, deep learning gained traction due to its remarkable ability to process and analyze large volumes of data with unparalleled accuracy.
Its rise to popularity can be attributed to significant advancements in computing power, the availability of vast datasets, and breakthroughs in neural network architectures. These factors have collectively fueled the widespread adoption of deep learning across various industries, including manufacturing.
In manufacturing, deep learning AI is revolutionizing traditional processes by enabling predictive maintenance, quality control, and automation. By leveraging sophisticated algorithms, deep learning systems can analyze sensor data in real-time to detect anomalies, predict equipment failures, and optimize production processes.
Furthermore, deep learning algorithms can enhance product quality by identifying defects with greater precision than traditional inspection methods. This capability not only reduces waste and production costs but also ensures compliance with stringent quality standards.
Overall, the integration of deep learning AI in manufacturing promises increased efficiency, improved product quality, and enhanced operational insights, thereby driving innovation and competitiveness in the industry.
Vision deep learning AI, also known as computer vision, operates by mimicking the human visual system to interpret and understand visual data. In general, there total of 4 steps, 1. Dataset capturing, dataset labeling 3. Training 4. Inferencing. But in slightly detail , Here’s a simplified explanation of how it works:
Data Acquisition: The process begins with acquiring visual data, typically in the form of images or videos, through cameras or other imaging devices.
Preprocessing: The raw visual data may undergo preprocessing steps to enhance quality, remove noise, or standardize formats. This step ensures that the data fed into the deep learning model is clean and standardized.
Feature Extraction: In this stage, the deep learning model extracts relevant features from the input data. Convolutional Neural Networks (CNNs), a common architecture used in vision tasks, excel at feature extraction. CNN layers consist of filters that convolve across the input image, capturing patterns and features at different scales.
Feature Representation: Extracted features are then transformed into a representation suitable for further processing. This representation often involves flattening or pooling the extracted features to reduce dimensionality while preserving important information.
Learning: The transformed features are fed into one or more layers of the deep learning model, where learning takes place. During training, the model adjusts its internal parameters (weights and biases) based on the input data and associated labels. This process involves optimization algorithms like stochastic gradient descent to minimize the difference between predicted and actual outputs.
Classification or Regression: After learning from the training data, the deep learning model can perform various tasks depending on the application. For classification tasks, the model assigns labels or categories to input images (e.g., identifying objects in images). For regression tasks, the model predicts continuous values (e.g., estimating the position of objects).
Evaluation and Testing: The trained model is evaluated using a separate dataset to assess its performance. Metrics such as accuracy, precision, recall, and F1-score are commonly used to measure the model’s effectiveness.
Deployment: Once the model demonstrates satisfactory performance, it can be deployed in real-world applications to analyze new, unseen visual data. Deployment may involve integrating the model into software applications, embedded systems, or cloud services, depending on the specific requirements.
Through this process, vision deep learning AI systems can perceive, interpret, and extract meaningful information from visual data, enabling a wide range of applications such as image recognition, object detection, facial recognition, medical imaging analysis, autonomous vehicles, and more.
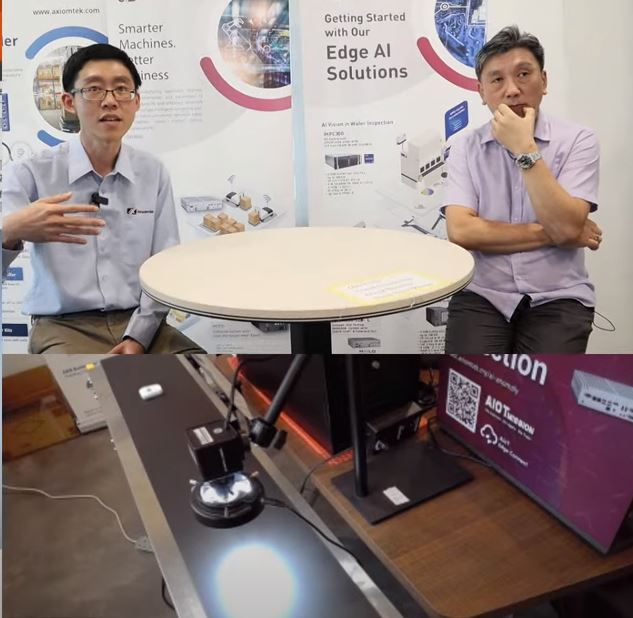
In this session, we demonstrated the Vision AI model with the the detection of Label sticker on a power plug simulated production. Those with label being good and no lable being NG.
The Camera is placed on top of the running conveyor with estimated 0.5 Meter / seconds. The camera is able to perform a the capturing speed of about 80 Frames per second. With the trained AI model, un the lighting control. Axiomtek AIS is able to detect and count the good and bad output.
The processed data from AI was then feed to the SCADA software and the SCADA software is presenting these set of Data live at the screen.
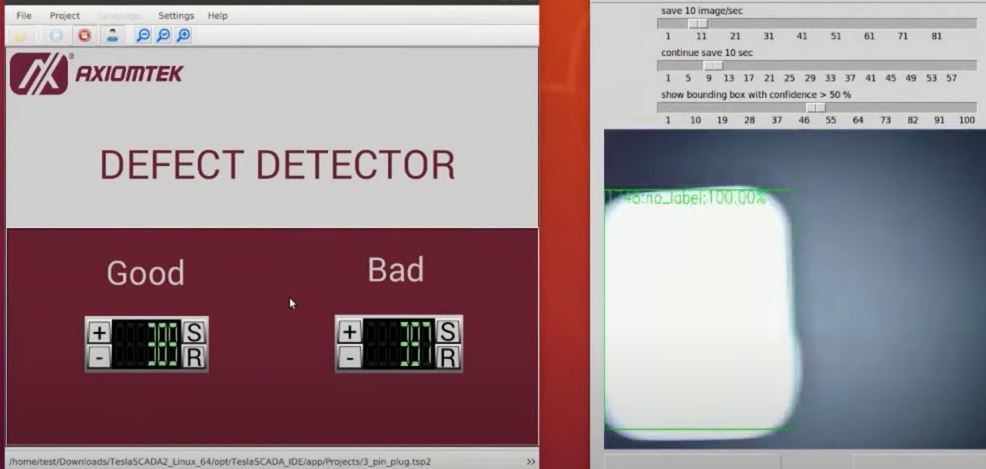
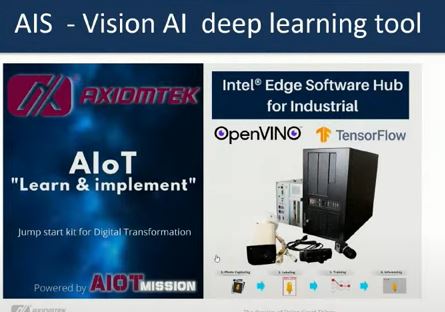
In order to complete the whole process of Vision inspections, here are the components needed: –
- Axiomtek Industrial PC
- AIS development software built-in in the IPC
- USB high speed camera
- the subject of inspection. In this case, it is the power plug
Features of the Axiomtek AIS :
● Deploy without programming: web apps design with simple and intuitive user
interfaces
● Intel® Edge Software Hub for Industrial: support both model optimizer and
inference engine by Intel® OpenVINO™
● End-to end deep learning: all-in-one implementation from training to inference
● Built-in dashboard components: simple dashboard components for users to easily
and quickly deploy training and inference
you may also read some related AI related post for optimizing smart manufacturing process with AI.
Watch the session at our youtube channel as below: Do share and subscribe if you think this is relevant.