” Sembang AIoT” live session was hosted by Axiomtek Malaysia again on the 6th of April 2024. In this session, AI Data Analytic for smart manufacturing was discussed in a greater depth where the challenges of applying AI data analytic was put on the table for discussion.
What could be the main challenges in the application of AI data analytic in Smart Manufacturing?
Data Quality and Availability: Manufacturing data may be noisy, incomplete, or of varying quality. Ensuring data accuracy and availability for training AI models is crucial.
Interoperability and Integration: Manufacturing environments often use diverse systems and equipment, leading to interoperability challenges when integrating AI analytics across different platforms.
Complexity of Processes: Manufacturing processes can be complex, involving numerous variables and interactions. Developing AI models that accurately capture and optimize these processes requires deep understanding and expertise.
Real-time Decision Making: Many manufacturing operations require real-time decision-making, which poses challenges for AI systems to analyze data, make predictions, and provide insights quickly enough to support operational needs.
Security and Privacy Concerns: Manufacturing data may include sensitive information about products, processes, or intellectual property. Protecting this data from cybersecurity threats and ensuring compliance with regulations is critical.
Scalability: Implementing AI analytics in large-scale manufacturing operations requires scalable solutions that can handle massive amounts of data and computational resources efficiently.
Human-Machine Interaction: Integrating AI analytics with human operators and decision-makers in manufacturing environments requires careful consideration of user interfaces, training, and acceptance of AI-driven recommendations.
Cost and ROI: Investing in AI analytics involves significant costs for infrastructure, talent, and implementation. Demonstrating a clear return on investment (ROI) and aligning AI initiatives with business objectives are essential for successful adoption.
Addressing these challenges requires collaboration between data scientists, engineers, domain experts, and stakeholders to develop robust AI solutions tailored to the specific needs of manufacturing processes.
IIoT Edge - Cloud - AI Data analytic - SCADA
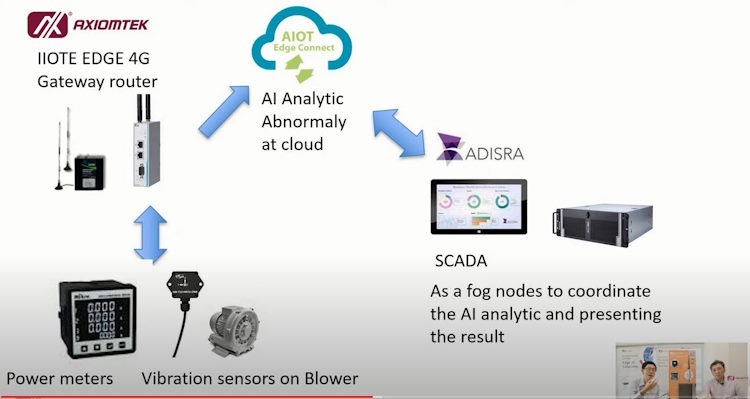
The live demonstration of AI Machine Learning comprises four main components: Sensors, Edge Gateway, Cloud Platform, and SCADA. Starting at the lowest level, data sources such as Modbus RTU-based Vibration sensors and Digital power meters capture information, which is then transmitted to the IIoT Edge gateway or 4G IoT Gateway router. These data are subsequently published to the cloud. While data analytics are conducted on the cloud platform in this demonstration, for enhanced efficiency, it is recommended that the analysis be performed by a local on-premise AI Engine with Cloud dashboard functionality. Presentation or reporting tasks are then handled by the SCADA system.
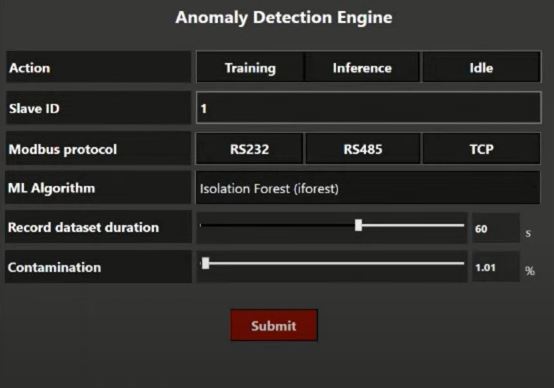
Anomaly Detection with AI Machine learning. The SCADA dashboard act source of control the data source of selection and triggering of the ML Training.
Inference result presented on the AdisRa SCADA with a Live Trending Chart of the results.
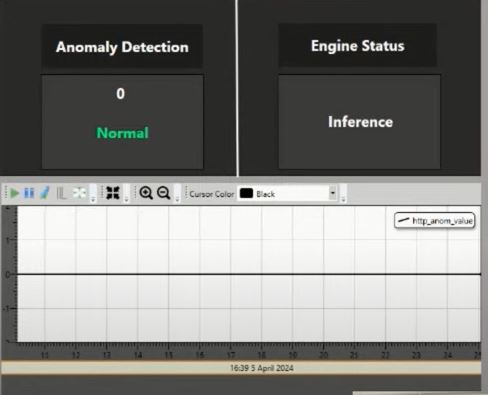
To watch this this live session please visit the link below and don’t forget to like ,share and subscribe if you find this relevant. Thank you.
https://www.youtube.com/watch?v=zwjjpBPL850&t=5s
Pingback: AIoT predictive Analytic for Smart Manufacturing - Axiomtek industrial PC Malaysia
Comments are closed.