Delving deeper into the Industrial Internet of Things (IIoT) within the context of Industry 4.0, Mr. CC Lee and Tan Kien Leong, distinguished technical experts from Axiomtek Malaysia and Accredited HRDC trainers at AIoTmission, recently conducted the thirty-eighth session of “Sembang AIoT.” This session delved into the foundational aspects of the Internet of Things (IoT) in the industrial sector, elucidating the pivotal role played by IoT in fostering Industry 4.0 and driving digital transformation. As primary suppliers of industrial computers and PCs, Axiomtek Malaysia is actively engaged in pioneering innovations in the realms of Artificial Intelligence (AI) and IoT applications, specifically focusing on industrial transformation.
Our live session in AIoT garnered an overwhelming response from our partners and followers. We extensively covered AIoT applications, spanning from the foundational levels to the most advanced stages of implementation and integration. As a token of appreciation, we extend our heartfelt thanks to everyone for their unwavering support. We trust that the insights shared during our session will prove valuable for your business endeavors.
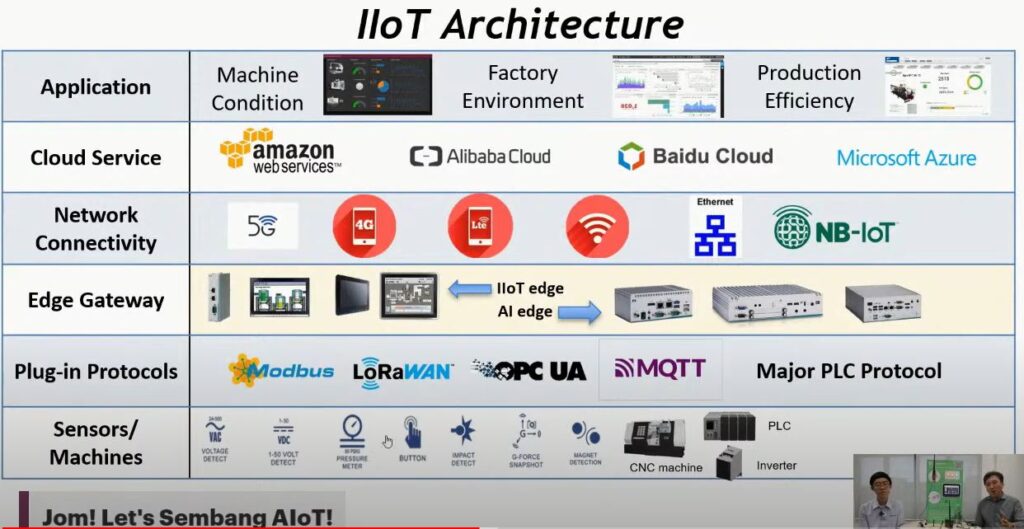
We discussed the IoT sensors and data collection method and in we shared the Industrial IOT architecture:-
The Industrial Internet of Things (IIoT) architecture encompasses multiple layers, from the low-level devices to the cloud and applications. Here’s a brief overview of the typical components at each level:
Device/Edge Layer:
Sensors and Actuators: At the lowest level, physical devices like sensors and actuators collect and interact with the real-world data in the industrial environment.
Edge Devices/Gateways: These devices serve as a bridge between the sensors and the upper layers, performing initial data processing and sometimes storing essential information locally.
At this Edge level, Axiomtek provides a complete IOT edge gateway and also the AI Edge embedded system to serve the whole IOT eco system.
Communication Layer:
Communication Protocols: Various communication protocols (e.g., MQTT, CoAP, OPC UA) enable seamless data transfer between edge devices, sensors, and the higher layers.
Control Layer:
Controllers: This layer includes programmable logic controllers (PLCs) or other control devices responsible for managing and controlling the connected devices in real-time.
Data Processing Layer:
Edge Computing: In some architectures, edge devices or gateways process data locally, performing tasks like filtering, aggregation, or even running simple analytics before transmitting relevant information to the cloud.
Fog Computing: Similar to edge computing, fog computing involves decentralized computing resources between the edge and the cloud, providing more processing power and reducing latency.
Communication Infrastructure Layer:
Networks: Robust and secure communication networks, such as Ethernet or industrial protocols, facilitate data transfer between different components within the system.
Cloud Layer:
Cloud Services: The data collected from the industrial environment is sent to the cloud for storage, analysis, and long-term processing.
Database Storage: Cloud databases store vast amounts of historical and real-time data.
Data Analytics: Advanced analytics tools process the data to derive actionable insights and support decision-making.
Machine Learning/Artificial Intelligence: In some cases, machine learning algorithms or AI models are employed for predictive maintenance, anomaly detection, and optimization.
Application Layer:
User Interfaces: Applications and dashboards provide a user-friendly interface for end-users, engineers, and decision-makers to visualize data and monitor the industrial processes.
Integration with Business Systems: Integration with enterprise resource planning (ERP) systems and other business applications ensures that insights derived from IIoT are aligned with overall business goals.
This architecture allows for a seamless flow of data from the industrial devices to cloud-based applications, enabling real-time monitoring, analysis, and optimization of industrial processes.
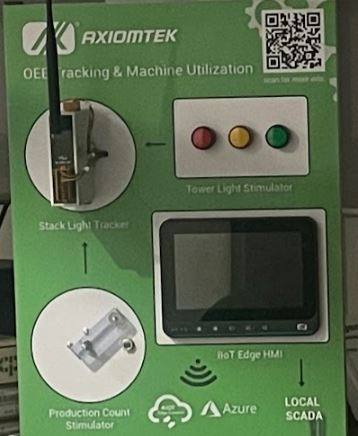
Demonstrated IOT OEE tracker for smart manufacturing. key components are Tower light ( stack light tracker) , IOT EDGE gateway and HMI. Cloud interface
Node red built on Node JS, a free open source IOT tools that allow users to built and deploy IOT project in a very cost efficient manner.
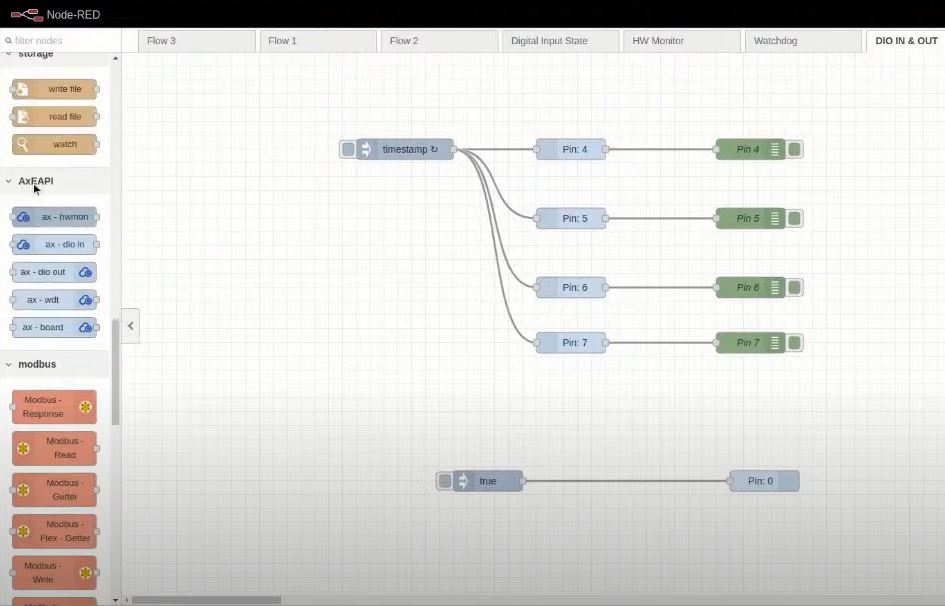
Predictive maintenance was used in the example in the this live session where IoT roles are highlighted.
Predictive maintenance in smart manufacturing is a key application of the Internet of Things (IoT), leveraging data from connected devices and sensors to predict when equipment or machinery is likely to fail. Here’s how IoT is used in predictive maintenance within the context of smart manufacturing:
Sensor Deployment:
Condition Monitoring Sensors: IoT-enabled sensors are strategically deployed on machinery and equipment to continuously monitor various parameters such as temperature, vibration, pressure, and other relevant metrics.
Edge Devices: Sensors are often connected to edge devices or gateways that preprocess the raw data locally before transmitting it to the cloud. This helps reduce latency and bandwidth requirements.
Data Collection and Transmission:
Real-Time Data Streams: The sensors collect real-time data, providing a continuous stream of information about the operational status and health of the equipment.
Connectivity Protocols: IoT devices use communication protocols like MQTT or CoAP to transmit the collected data securely to cloud platforms.
Cloud-Based Analytics:
Cloud Services: The data is sent to cloud-based platforms equipped with advanced analytics tools, machine learning algorithms, and artificial intelligence capabilities.
Data Storage: Cloud databases store historical and real-time data, facilitating analysis and pattern recognition.
Data Analysis and Predictive Algorithms:
Machine Learning Models: Advanced machine learning models are applied to the collected data to identify patterns, correlations, and anomalies.
Predictive Algorithms: Predictive maintenance algorithms use historical data to predict when equipment is likely to fail, estimating the remaining useful life of critical components.
Alerts and Notifications:
Threshold Monitoring: IoT systems set predefined thresholds based on normal operating conditions. If the monitored parameters deviate from these thresholds, the system triggers alerts.
Maintenance Recommendations: Predictive maintenance systems provide actionable insights, recommending specific maintenance actions to be taken to prevent unplanned downtime.
Integration with Maintenance Workflows:
Enterprise Integration: The insights derived from predictive maintenance are integrated with enterprise resource planning (ERP) systems and maintenance management workflows.
Work Order Generation: Predictive maintenance systems may automatically generate work orders for maintenance teams, specifying the required actions and scheduling.
Benefits:
Reduced Downtime: By predicting potential failures in advance, organizations can schedule maintenance activities during planned downtime, minimizing disruptions to production.
Cost Savings: Predictive maintenance helps optimize maintenance schedules, reducing unnecessary maintenance and minimizing the need for emergency repairs.
Increased Equipment Lifespan: Proactive maintenance based on IoT insights can extend the lifespan of equipment and machinery.
In summary, IoT plays a crucial role in predictive maintenance by providing real-time data, leveraging advanced analytics, and enabling proactive decision-making to optimize maintenance processes in smart manufacturing environments.
To watch this session live click at the link below:-